Instead of requiring users to build queries, maintain dashboards, or manually transform data, agentic analytics shifts the responsibility to intelligent systems that handle those tasks for you—accurately, transparently, and repeatably.
This isn’t about a chatbot summarizing numbers. It’s about AI understanding how BI actually works—query structure, data models, aggregation logic, visual rules—and executing each of those steps using a deterministic analytics stack.
{{Call Out Component}}
Why Agentic Analytics Matters
Traditional analytics tools weren’t built for this. The modern data stack is fragmented—data prep happens in one tool, querying in another, visualizations in a third. That system was designed for teams of specialists, not for intelligent agents.
Agentic Analytics flips that model. It requires an integrated environment where an agent can seamlessly move from ingesting and transforming raw data to structuring visuals and summarizing insights.
The result? A system that doesn’t just mimic human analysts—it performs the work itself. Every calculation, transformation, and visualization follows structured, repeatable logic. You can see the filters, the formulas, and the rules—so you know exactly how the data is being analyzed.
“People don’t want the feeling of their numbers. They want the actual numbers—calculated correctly, structured cleanly, and interpreted in context.”
What Makes Analytics Agentic?
Agentic Analytics™ isn’t a single feature—it’s a full stack approach. The AI agent isn’t just giving you an answer—it’s driving the infrastructure to get that answer the right way.
Here’s how it works:
1. Semantic Understanding of Data
When data is ingested, the system uses high-level reasoning to understand what it’s looking at. It builds a semantic model of the dataset—identifying fields, relationships, and patterns automatically.
2. Automated Data Preparation
Before any analysis happens, the system transforms the data into a usable analytical model—creating derived fields, bucketing values, building date-based segments, and structuring the dataset for effective analysis.
Think: converting timestamps into morning/afternoon/evening categories or turning zip codes into geographic regions—work analysts usually do manually, now handled by the agent.
3. Deterministic BI Execution
The agent then drives the actual BI tool—applying filters, building aggregations, selecting metrics, and generating visualizations using structured rules, not approximations. These are not one-off summaries. They're reusable, structured artifacts that update as data changes.
4. Narrative Assembly
The system doesn’t just output charts—it assembles them into coherent data stories. The agent acts like an analyst, deciding what to highlight, how to group insights, and how to present them in a meaningful way for business users.
5. Integrated Advanced Analysis
Agentic Analytics extends beyond traditional BI. It also incorporates machine learning models, applying statistical methods and surfacing patterns that human analysts often miss. The agent interprets these results and packages them into understandable summaries.
“A typical output in Scoop might involve 100+ agent instructions behind the scenes—each directing a discrete action by the BI tool. The result is a structured, narrative-ready analysis delivered without manual effort.”
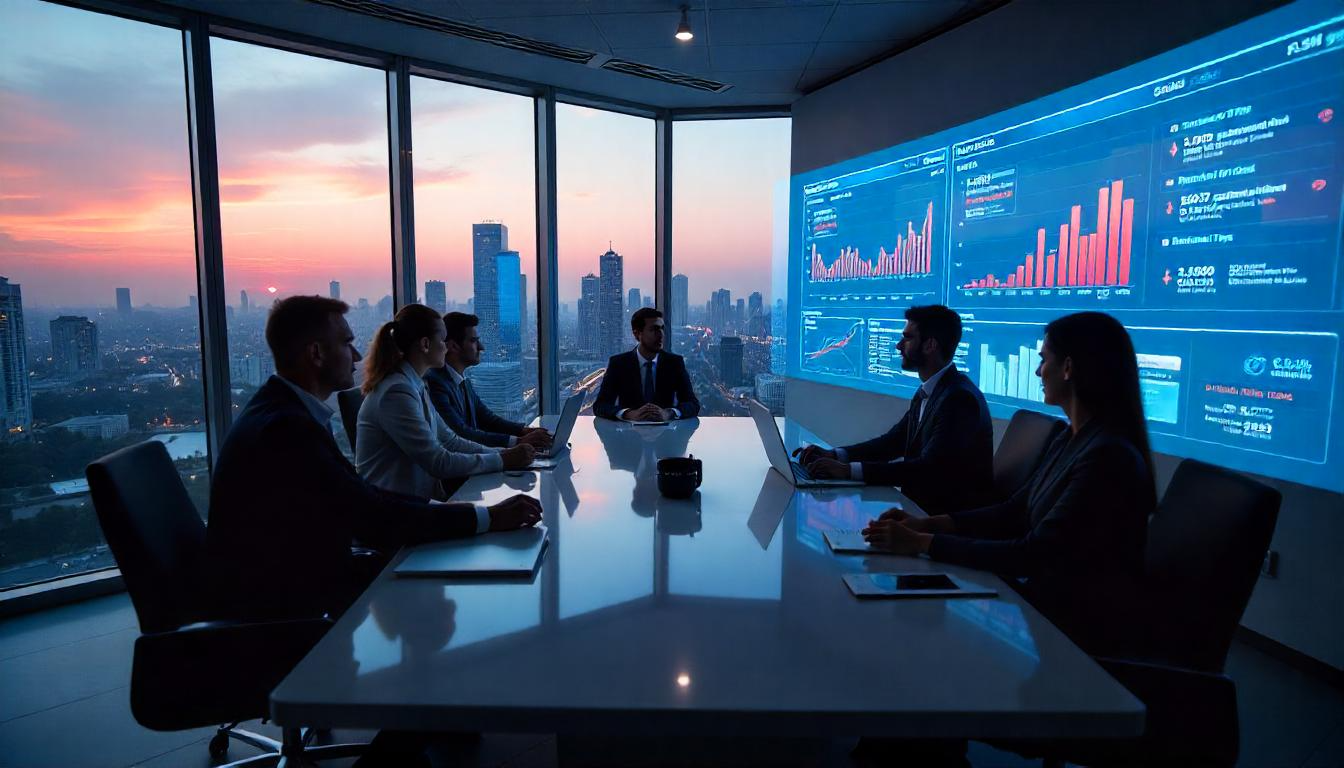
How Agentic BI Fits In
Agentic BI is a specific application of this broader concept. It refers to how an AI agent automates traditional BI workflows—reporting, dashboards, metrics, and chart creation.
But Agentic Analytics goes further. It combines data prep, BI, advanced analysis, and interpretation under one system. It’s not just about simplifying BI—it’s about rethinking how data work gets done altogether.
“Agentic BI is how the agent runs your dashboards. Agentic Analytics is how the entire stack—data prep, BI, and machine learning—is orchestrated for you.”
Why Most Tools Can’t Deliver It
Most analytics platforms can’t support agentic workflows—not because AI isn’t capable, but because the infrastructure isn’t. Disconnected tools can’t be operated end-to-end by an agent. You need an integrated system—one where data, structure, and visualization are all connected and controllable.
“If your charting engine is different from your data engine is different from your prep engine, an agent can’t operate it. Those stacks were built for people—not for agents.”
Agentic Analytics requires more than an AI overlay—it demands a rethinking of how analytics systems are built and used.
Final Thought
Agentic Analytics isn’t a buzzword—it’s a shift. A new model for how data work gets done. One where AI agents handle the operational complexity, so people can focus on decisions, not dashboards.
It’s not just about getting answers faster—it’s about getting better answers, delivered through systems you can trust, interpret, and reuse.
If BI was the first wave of self-service analytics, Agentic Analytics is the next one—and it’s built to work the way modern teams actually need.